Parallel optimization testing
Testing approach
While carrying out the testing of the developed algorithm, a number of
double-criteria optimization problems have been solved utilizing different numbers of
slave processors (CPUs): (NCPU= 1, 5, 10, 20, 30, 40). Problems
of different dimensionalities (Nx= 20, 40, 60, 80) have been solved for
test functions:


The analytical determination of the Pareto set for the given test is
extremely difficult. The "exact" solution has been obtained by means of the
detailed scanning of variable parameters space (the scanning interval had the value of 10-4)
for Nx=2. It is easy to realize that the increase of the optimization
problem dimensionality (Nx=4,6,8,) would not lead to the
"exact" solution change.
To carry out the numerical testing of the multicriteria optimization
algorithm it is necessary to introduce some quality index which is being obtained as a
result of the Pareto set. It is common to use the solution mean error, defined as
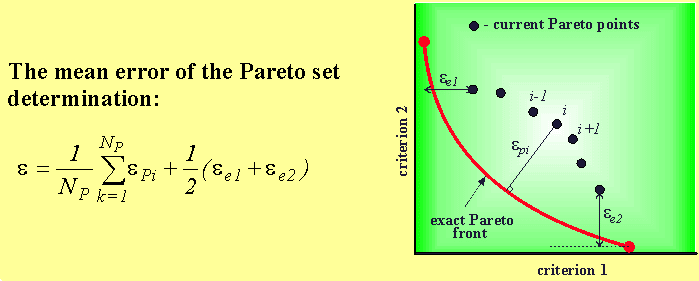
The optimization efficiency assessment for the use of parallel processors
was carried out using the following indices:
It must be noted that when using traditional approach to the
analysis of parallelization computation efficiency the ideal value of this index is equal
to the number of the applied processors (SN=N). However, for the
parallel optimization algorithm, the change of the number of processors being used
corrects the information volume which is being analyzed when going from one iteration to
another. Thus, in our case the effectiveness of computations parallelization (SN
/N) can be both less and more than 1.
The testing results.
This figure shows an example of SN
parameter variation demonstrating the high parallelized computations
efficiency. One can see that for the considered test conditions the
SN parameter value exceeds the total number
of operational CPUs. It proves that this parallel optimization
algorithm has the higher "internal" efficiency than one
of IOSO basic (single-CPU) version. It also proves that the given
optimization procedure has been developed according to the parallel
optimization process, and that it does not represent a trivial usage
of several CPUs for the solution of optimization problems. |
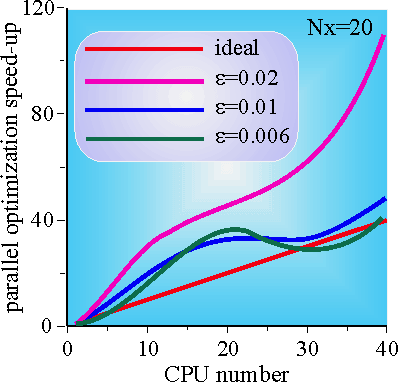 |
|